Integration of AI With Emerging Technologies for Environmental Work
Posted: January 11th, 2024
Authors: Aditya S. Teck L.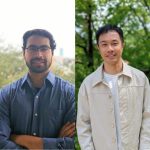
In today’s rapidly evolving world, the role of technology, particularly artificial intelligence (AI), has become increasingly pivotal across various sectors. Environmental professionals tasked with ensuring regulatory compliance, improving air quality, enhancing environmental, health, and safety (EHS) standards and advancing Environmental, Social, and Governance (ESG) frameworks stand to benefit significantly from integrating AI-powered solutions.
Machine Learning (ML), Natural Language Processing (NLP), Robotic Process Automation (RPA), and Image Recognition are poised to revolutionize the landscape of environmental compliance, offering unprecedented capabilities to address complex challenges.
Machine Learning and Environmental Compliance
Machine Learning algorithms are at the forefront of transforming environmental compliance processes. These algorithms excel at analyzing vast datasets, identifying patterns and predicting potential noncompliance issues. For instance, ML models can analyze historical data related to regulatory violations, emissions data, monitor reliability or environmental impact assessments to forecast potential risks accurately. Here are some examples of emerging AI use that can empower facilities to proactively address compliance issues before they escalate and to deploy better strategies to operate efficiently:
Scenario Modeling and Simulations
Scenario modeling and simulations are invaluable tools for navigating environmental compliance and permitting. They involve creating detailed simulations to predict the environmental impact of proposed projects or operations under various conditions.
If you are an industrial manufacturer planning to expand operations or install a new facility, then scenario modeling can help you assess the impact of emissions, resource usage and compliance costs. It can simulate the effects of different fuel inputs, operating capacities, waste treatment methods or the introduction of cleaner production technologies, providing you with a better understanding of potential compliance obligations and regulatory risks.
Forecasting Maintenance Schedules With ML Models
ML models are increasingly used to assess equipment performance and predict component degradation or failure, allowing facilities to forecast maintenance schedules and optimize resources for equipment upkeep. The ability to proactively address equipment/process issues reduces cost, improves uptime and mitigates regulatory risks, providing a competitive edge to businesses. There is a growing demand for developing statistical models that can predict sensor degradation or calibration drift of Continuous Emissions Monitoring Systems (CEMS), which are used for compliance demonstration.
At facilities with CEMS, failure to monitor emissions continuously may result in a deviation, forcing the operators to shut down to avoid further violations. Unscheduled or abrupt shutdowns lead to production losses and adversely affect process equipment. A simple predictive system developed with input from domain experts and ALL4 has helped facilities identify potential CEMS failures or excessive drift beforehand, allowing operators to initiate prompt corrective action and minimize noncompliance.
Monitoring Process Variable Using Soft Sensors
Soft sensors — computational models that can estimate process variables — are being tested and deployed in water and wastewater treatment plants to monitor and optimize various parameters without relying solely on hardware sensors. These sensors are developed using hardware sensor data to calibrate a mathematical model, which outputs a certain target parameter, such as water quality estimation or chemical dosing requirements.
The benefits of using soft sensors in wastewater treatment plants include cost-effectiveness, as they provide a low-cost alternative to hardware sensors and real-time data, unlike laboratory sampling, which has inherent delays.
Addressing Environmental Challenges With New AI Tools
Regulatory agencies, advocacy groups and the public are actively exploring new AI tools to address environmental challenges better. The U.S. Environmental Protection Agency (EPA) has been assessing the utility of ML tools to identify violations, support facility inspections and enhance enforcement targeting. Applying these techniques at your facility can help identify and address potential risks, minimizing the likelihood of agency inspection.
There is a growing adoption of advanced computational methods in environmental rule-making, specifically in monitoring emissions and modeling risk exposures. For instance, the recent EPA regulation aimed at reducing methane emissions from the Oil and Gas industry discusses using simulation-based methods and satellite data for emissions detection and leak mitigation.
When a state-specific reasonably available control technology (RACT) rule with new emissions standards was implemented, it was unclear for affected facilities whether they could meet the new standards or would require additional emissions control. To navigate the uncertainty, many facilities worked with ALL4 to perform ‘what-if?’ analyses to understand their ability to comply with the new standard and identify certain operating scenarios that may not meet the standards. Based on these analyses, facilities could confidently develop and justify suitable compliance strategies and stay ahead of the industry.
AI for Environmental Monitoring
Environmental monitoring is vital for tracking the health of ecosystems, air and water quality and ensuring compliance with environmental regulations. However, traditional methods, such as manual sampling, laboratory analysis and in-field observations, face several limitations. These methods are often time-consuming, costly and prone to human error.
They do not provide real-time data, leading to delays in addressing environmental concerns like pollution or natural disasters. The manual nature of data collection also restricts the scale and scope of monitoring efforts, particularly in regions with limited resources or in remote areas.
Potential AI-Based Solutions
AI has the potential to transform environmental monitoring by addressing the inefficiencies of conventional methods. With AI, monitoring can become more precise, scalable and real-time. Machine learning (ML) algorithms and deep learning (DL) models enable the analysis of large datasets, identifying trends and patterns that would be impossible for humans to detect manually. Some practical scenarios of environmental monitoring with AI include:
- Air quality monitoring: AI systems are revolutionizing air quality monitoring by enabling continuous, real-time tracking of pollutants like particulate matter, nitrogen dioxide (NO₂) and carbon monoxide (CO). Through data from sensors and satellite imagery, AI models can detect pollution hot spots, track pollutant movements and predict air quality levels for specific locations.
- Water quality tracking: AI enables continuous water quality assessments using sensors deployed in rivers, lakes or coastal areas. Machine learning algorithms can analyze data on parameters such as pH, dissolved oxygen, turbidity and temperature to identify trends and predict contamination events, such as chemical spills or harmful algal blooms.
- Soil monitoring and land analysis: Agricultural sectors and conservation groups are increasingly using convolutional neural networks (CNNs) to assess soil composition from aerial images. These tools help optimize irrigation, predict yield and flag soil erosion risks with greater precision.
- Natural disaster prediction: Natural disasters such as earthquakes, hurricanes and wildfires cause significant damage to ecosystems and human populations. Traditional disaster prediction methods rely on limited data and can only offer predictions with a considerable time lag. AI enhances disaster prediction by analyzing real-time data from various sources, including satellite images, seismic sensors and meteorological data. AI models can detect subtle environmental changes that might indicate an impending disaster, providing early warnings to affected areas.
How AI Is Transforming Environmental Conservation
AI is playing an increasingly important role in conservation efforts by offering innovative tools to monitor biodiversity, protect endangered species and preserve natural habitats. Traditional conservation methods often struggle with challenges like limited data, remote locations and the need for long-term monitoring. AI helps overcome these challenges by providing real-time, scalable solutions covering large geographic areas and diverse ecosystems.
Examples of transformative AI use cases in environmental conservation include:
- Wildlife detection and tracking: AI algorithms trained on drone and trail cam imagery now support species recognition, animal counting and migration tracking across protected areas. These systems help conservationists monitor endangered populations without intrusive human presence.
- Forest health assessment: Using satellite images, AI models detect tree canopy stress and illegal logging activities in near real time. Combined with GPS data, these tools support targeted interventions and policy enforcement in remote regions.
- Marine conservation: AI-driven acoustic analysis helps identify illegal fishing activities by detecting motor signatures in protected waters. Meanwhile, deep learning models monitor coral bleaching events by analyzing underwater footage and ocean temperature data.
AI System as BACT?The Clean Air Act mandates using the best available control technology (BACT) to set air pollution limits, which traditionally focuses on physical devices like scrubbers and catalytic oxidizers. However, AI systems are emerging as a powerful tool in this domain. They can rapidly analyze vast amounts of data to optimize production processes and the operation of pollution control equipment. Effectively utilized, AI can enhance the efficiency of these systems, reducing the pollution generated and emitted into the environment. As AI technology advances and gets integrated into more systems, will it become recognized as BACT? Can it eventually become a standard or even a requirement under the regulations of the Clean Air Act? |
Natural Language Processing for EHS Management
Natural Language Processing, a branch of AI focused on understanding and processing human language, holds immense promise in revolutionizing EHS management in environmental consulting. NLP-powered systems can interpret unstructured textual data from reports, surveys, or regulatory documents, enabling environmental professionals to extract critical insights and trends efficiently. For example, they can:
- Read, summarize, and translate environmental regulations,
- Track changes in the regulations and developments from government policy-making,
- Mine regulatory dockets to see how the public commented on specific regulations or proposed regulations,
- Recommend action plans and tasks for compliance with external and internal obligations.
Companies are already utilizing NLP systems to analyze and compare the permit conditions of their peers and competitors. This approach helps evaluate the relative stringency or leniency of their permit conditions. By gaining insights from this comparison, companies are better equipped to develop more effective permitting strategies.
Enterprise AI tools like ChatGPT and Microsoft Copilot, and their integrations across various products (Excel, Power BI, and Word), offer functionalities like:
- Using AI-powered assistance in data cleaning, normalization, and transforming raw data into a suitable format for analysis.
- Leveraging AI algorithms to automatically detect patterns, trends, relationships and anomalies within the data without explicit user queries.
- Allowing users to ask questions or make queries using natural language, and using AI to interpret these queries and generate relevant visualizations or responses.
- Utilizing AI to recommend suitable visualizations based on the data type and the analysis being performed.
AI tools can quickly scan through large volumes of text, identify key points and summarize information, making the review process more efficient. These tools can also check for consistency in terminology and flag potential errors or ambiguities, enhancing the accuracy of your documents or submittals.
Robotic Process Automation for Streamlined Reporting
Robotic Process Automation (RPA) plays a vital role in automating repetitive tasks involved in reporting. RPA tools can extract data from disparate sources, compile reports and even assist in generating reports based on different regulatory frameworks and reporting requirements, thereby saving time and reducing human errors.
Examples of RPA applications are the extraction of digital files from emails/folders, automation in filling forms (web forms/electronic files), and submission of completed forms. RPA uses multiple tools and software to perform these tasks automatically or when an event is triggered.
A specific use case is gathering ESG-related data for reporting to different reporting frameworks. ESG encompasses a broad spectrum of metrics, from carbon emissions and energy usage (Environmental) to employee diversity and community relations (Social) to board diversity and business ethics (Governance). Collecting data on these diverse factors requires different methodologies and sources.
In addition, obtaining reliable and comprehensive data can be challenging. Some relevant data might not be publicly available, or companies might not have systems to consistently collect and report specific ESG metrics. Moreover, data accuracy and consistency over time add another layer of complexity. An RPA allows ESG specialists to focus on interpreting insights rather than gathering information and spending time evaluating the accuracy of the data.
ALL4 Automated State-Specific Emissions Reporting Tool (ASSERT) is a reporting automation application developed by ALL4 industry experts and developers. The tool extracts data from Excel spreadsheets and produces files (e.g., CSV, XML and JSON) containing emissions data compatible with state emissions inventory reporting systems.
It performs state-specific data quality checks and generates an error report that provides the location and context of data that did not validate against state-specific criteria. It then produces files that may be readily imported into the state’s system, such as the State and Local Emissions Inventory System (SLEIS), supported by numerous states nationwide.
Image Recognition AI and Air Quality Monitoring
AI-powered image recognition coupled with advanced sensors and cameras can transform health and safety and air quality monitoring. Image Recognition AI can process vast amounts of data, including surveillance videos, climate models, satellite imagery and historical records. It can help identify unsafe work practices and predict environmental changes, like climate patterns, deforestation rates or biodiversity shifts. This predictive capacity allows facilities to better anticipate and plan for environmental impacts.
For example, a city can utilize Image Recognition AI to monitor air quality by analyzing images captured by drones equipped with specialized sensors. This real-time analysis aids in promptly identifying pollution sources and implementing targeted mitigation measures.
Current Progress and Future Prospects
While significant strides have been made in implementing AI across environmental domains, the future holds even more promise. Ongoing research and development efforts are aimed at enhancing the capabilities of AI in this field.
- Hybrid models: Integrating multiple AI technologies to create hybrid models for comprehensive environmental assessments.
- Predictive analytics: Advancements in ML for more accurate predictions of environmental risks and trends.
- Explainable AI: Development of AI models that provide transparent and understandable insights, crucial for decision-making in compliance and sustainability strategies.
Despite the potential benefits, integrating AI into environmental compliance comes with various challenges and ethical considerations. Privacy concerns, biases in data and reliance on AI without human oversight are critical issues that need to be addressed. Maintaining a balance between technological advancement and ethical use remains imperative.
Harnessing AI to Empower Environmental Professionals and Initiatives
At ALL4, we are exploring the potential of AI to enhance our operations and work products. ALL4 recognizes that our greatest strength lies in our people. As part of ALL4’s AI initiative, we are gathering insights into our collective knowledge and experience with AI and identifying areas for further learning and development.
We are committed to exploring AI as a tool that empowers, not diminishes, our human capabilities. We view AI as a partner in our progress, not as a replacement for the ingenuity and skills that our people bring to our organization. As technology advances, the synergy between AI and environmental professionals will drive more efficient, sustainable, and ethical practices.
If you’re exploring how to integrate AI into your environmental work or have any questions about our AI initiatives, contact us today.